Behavioral Biometrics vs. User Behavior Analysis
The interest in behavioral biometrics has obviously grown in recent years and has grabbed the attention of marketers, security professionals, and investors alike and so too have the myths and expectations of behavioral biometric implementations. One clear line to draw to help define the role behavioral biometrics plays in a security system is to separate it from user behavioral analysis. While behavioral biometrics looks at the way a user interacts with a device, like mouse motions, keystroke dynamics, or touch screen interactions, the behavioral analysis looks at what a user interacts with in a web service and gives context to the actions they take.
Behavioral Biometrics: Mouse Event
Looking at the actual signals collected from behavioral biometrics can reveal strong differences between simple automated systems and real human users. The plots below show a reconstitution of how a real user (on the left) interacted with the page using the mouse, compared to synthetic events sent by a bot. The reconstitution is based on the X, Y coordinates collected through JavaScript at various intervals. Human behavior is usually complex and chaotic whereas automated behavior is simple and restricted to what is strictly necessary to complete a task:
In web security, looking at where the user moves his mouse or clicks is actually not the most revealing. Looking at the x-coordinate of the mouse cursor on a page versus time can help differentiate the human from the bot. With human-mouse motions (on the left), coordinate paths are often inconsistent, have clusters of points, and have “smooth” curves when changing position. When comparing the plot on the right of a simple automated program controlling the mouse on the same web interface, many discontinuities can be seen, and oftentimes, the time between each event is evenly spaced out. In this particular case, the bot doesn’t send any mouse move events and only sends click events.
Even more, differences become apparent when examining the velocity of the same mouse trajectory. With human-mouse velocity many inconsistencies are apparent and many sharp increases in the speed of the mouse motions can be seen over time. With basic automated systems, the velocity of the cursor is often linear in between movements and it is much less variable than human motion.
The acceleration profile of the mouse motion can further reveal more linear behavior with automated systems and greater variability across time with authentic human-mouse control. This same type of analysis can be applied to any form of interface control from the speed of keypress events to the patterns of touch screen interactions and all these dimensions can be combined to create a rich feature set for fraud detection.
The Benefits of Behavioral Biometric Detection
For account takeover, web scraping, and fake account creation, the events collected from behavioral biometrics can help detect these attacks in a way that is independent of IP or device information so when each dataset is combined it leads to a much stronger detection signal. For these same types of attacks, user behavioral analysis can help define custom rules on what type of behavior is expected or acceptable given the user’s actions during a session, the time of day, or how other users have behaved in the past.
While a formal behavioral biometrics implementation can be data-intensive and computationally expensive, these costs can be reduced by only sampling high information events instead of blindly collecting all the user interactions on a website. In a proper implementation, no personally identifiable information is collected during the sampling of these user interactions and no passwords or usernames are collected during any sampling of data.
The benefits of behavioral biometrics depend on the use case, but for bot and human fraud detection, the fact that all these events are passively collected is beneficial for both users and web owners. Users benefit since they can perform their normal activities on a web service with a lower chance of having security measures like Captchas or rate limits imposed on them, and web owners benefit because their service is more secure with less impact on its user experience. This passive interface helps reduce friction during the checkout process, account creation process, and streamlines the login flow for returning users to the service.
Another key benefit of behavioral biometrics lies in the difficulty to spoof the data itself. All information collected from client-side events can be spoofed, and behavioral biometric data is no exception. The difference lies in the difficulty and sophistication required to accurately falsify rich human interaction data versus simply spoofing something like a user agent. It takes time, resources, and knowledge of programming and data-savvy techniques to efficiently spoof behavioral biometric data at scale. That being said, if there is an incentive to falsify and spoof this data, it will happen, and new methods to identify this will be developed, but for now, at Arkose Labs the bar is being raised as we continue the cat and mouse game.
To learn more about Arkose Labs’ approach to behavioral biometrics, schedule a demo today.
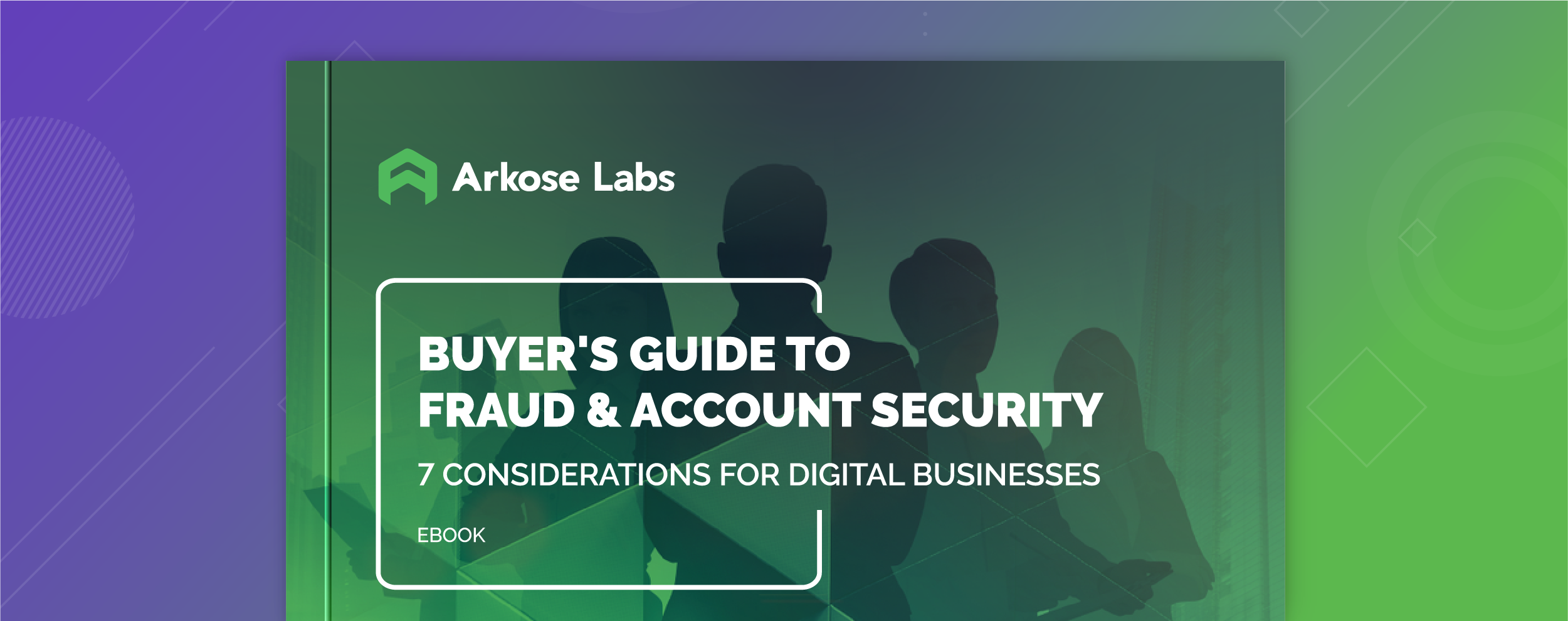